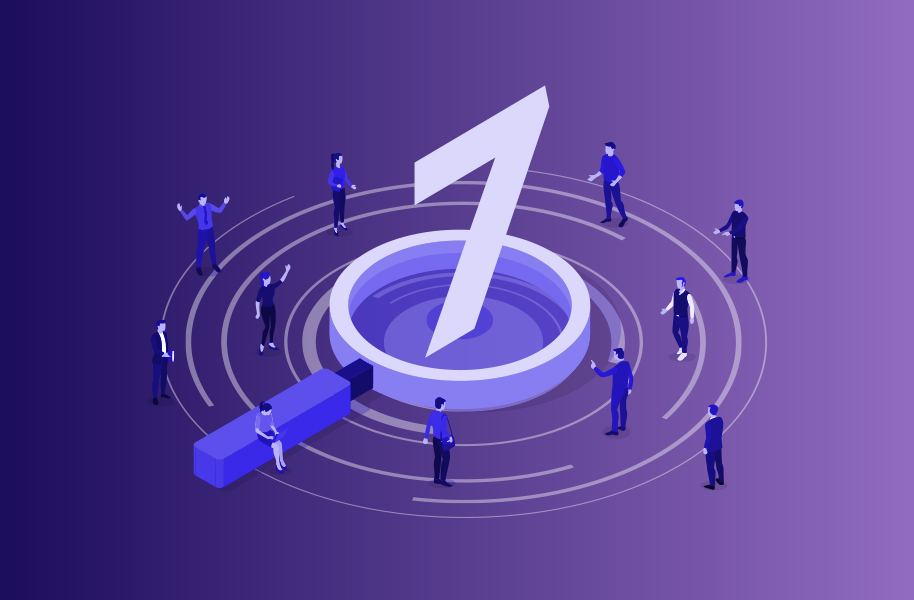
7 Customer Data Analysis Mistakes to Avoid
Customer data Analysis resembles pieces of a large puzzle. Individually, each piece holds simple information that may appear insignificant. Yet, when these pieces are combined and the relationships between them are analyzed, the entire picture emerges. Essentially, it acts as a guide for comprehending customer behavior and enhancing decision-making for customer service and satisfaction.
However, what happens if the analysis of this data goes awry? Without a doubt, incorrect analysis can result in erroneous decisions and the squandering of resources.
In this article, we will examine seven mistakes to avoid when analyzing customer data. If you seek to enhance your capacity to make informed decisions based on a precise understanding of your customers, ensure not to overlook this article.
Importance of Data Analysis
In recent years, there has been a significant surge in the volume and diversity of available data, underscoring the growing importance of data analysis and its applications across various domains. The importance of data analysis can be understood through several key aspects:
- Gaining Deeper Customer Insight
Failure to understand customers properly hinders a company’s ability to meet their needs, potentially resulting in the loss of customers to competitors. Data analysis plays a crucial role in illuminating customer preferences, needs, and behaviors, including browsing and purchasing patterns.
Subsequently, companies can tailor products or services to align with these insights. The deeper an organization’s understanding of its customers and their preferences, the better equipped it is to enhance customer loyalty, satisfaction, and ultimately increase sales.
- Creating Targeted Marketing Strategies and Campaigns
By leveraging data, companies can develop and implement targeted marketing campaigns aimed at reaching the appropriate audiences. This strategy relies on analyzing customer trends, monitoring online shopping behaviors, and assessing transaction data from points of sale.
Such practices enable marketers to craft personalized advertisements tailored to specific target segments, thereby enhancing the efficacy of marketing initiatives. Ultimately, this approach aims to surpass customer expectations and foster brand loyalty.
- Improving Operational Efficiency and Reducing Risks
The significance of data analysis extends to enhancing operational efficiency. How? Through the aggregation of extensive customer data and feedback, organizations can generate innovative concepts to enhance their offerings. Moreover, data analysis facilitates the identification of opportunities for operational streamlining, cost reduction, and profit maximization.
Furthermore, businesses can utilize insights from data analysis to promptly discern high-performing processes and areas of underperformance. Consequently, this informs strategic adjustments, preemptive problem-solving, risk management, and proactive enhancements.
- Exploring Opportunities for Innovative Products and Services
Data analysis empowers companies to comprehend their target audience, foresee and pinpoint product or service gaps, and subsequently innovate new offerings to fulfill these needs. Additionally, data analysis enables companies to refine their existing products or services in response to evolving consumer demands and enhance customer services.
7 Data Analysis Mistakes to avoid
In the realm of graphic analysis, avoiding common mistakes is crucial to maintaining the accuracy and effectiveness of the analysis. The success of any customer data analysis strategy hinges on the precision and consistency of the process.
In the subsequent paragraphs, we will outline seven common mistakes to steer clear of when analyzing customer data.
1. Using Low-Quality Data
Utilizing low-quality data yields a spectrum of negative effects, including:
- Inaccurate Analyses
Poor data quality fosters misinterpretation, resulting in flawed decision-making and subpar strategies.
- Missing Opportunities
Substandard data obstructs the identification of valuable leads, market trends, and customer insights, constraining business expansion.
- Damage to the Company’s Reputation
Employing poor-quality data may tarnish a brand’s image, eroding trust between customers and partners.
- Wasting Money and Financial Losses
Rectifying errors and addressing issues stemming from low-quality data incurs additional expenses, adversely impacting profits. On average, companies suffer approximately $15 million in annual losses due to inefficiencies and missed opportunities attributed to low-quality data.
- Customer Dissatisfaction
Inaccurate, low-quality data fosters unsatisfactory customer interactions, undermining loyalty and retention efforts.
2. Failure to clearly define goals and analyze unnecessary data
A clear definition of objectives and thorough analysis of necessary data form essential components of the customer data analysis process. Failure in either aspect can adversely impact the effectiveness of customer analysis, resulting in suboptimal decisions.
When goals lack clarity, the data analysis team may expend significant time analyzing irrelevant and unnecessary data, leading to resource wastage.
Moreover, ambiguous goals impede the attainment of accurate and valuable results from the data analysis process. Irrelevant data may yield incorrect conclusions and misleading decisions.
Furthermore, analyzing unnecessary data yields results of limited utility in making informed decisions concerning customers, products, and services.
Hence, it is paramount to establish specific goals before commencing customer data analysis and to focus solely on pertinent data to efficiently achieve those objectives.
3. Ignoring Bias in Data Analysis
Data bias refers to errors or systematic inaccuracies within a dataset, potentially leading to incorrect or unfair predictions during analysis or decision-making processes. The presence of bias can significantly impact the analysis of customer data in various ways, including inaccurate or incomplete comprehension of customers, markets, or business processes, resulting in ineffective marketing campaigns, imprecise customer targeting, and missed business opportunities.
Conversely, discovering bias within the data may damage a company’s reputation, provoke negative perceptions from the public, and even prompt legal actions, fines, and penalties against the organization.
To mitigate the adverse effects of data bias on customer data analysis, companies must actively strive to reduce bias at every stage of the data process. This involves ongoing evaluation and awareness of bias, leveraging multiple data sources to validate findings, and advocating for fairness and transparency throughout the analysis process.
Moreover, recognizing and mitigating common types of data bias such as sample bias, confirmation bias, observer bias, measurement bias, and reporting bias is crucial to upholding the integrity and dependability of analyses and decision-making procedures.
4. Misinterpreting Context of Data
Data Context encompasses the information and circumstances surrounding a dataset, shaping its meaning and significance. This includes elements such as data sources, purpose, collection methods, duration, and participant or entity details. What are the implications of not grasping the context of data?
Failure to understand the context of data invariably leads to erroneous conclusions about customer behavior and needs. For instance, a high purchase rate might suggest customer satisfaction with a product or service, but the actual reason could be a short-term marketing campaign.
Data collected devoid of context can yield misleading outcomes. For example, the average customer age may appear as 35, masking distinct groups—a cohort in their twenties and another in their fifties—leading to flawed conclusions and action recommendations.
Furthermore, lacking data context results in ineffective decisions regarding marketing strategies, product development, or customer service. A company might invest in a campaign targeting the wrong age demographic or launch a product misaligned with customer requirements.
Analyzing data without clear context consumes more time compared to analyzing contextualized data, squandering resources on futile analyses. Hence, gathering comprehensive information about data sources and surrounding factors is crucial to discerning the complete picture and the appropriate context.
5. Small Sample Size
One common mistake in customer data analysis is relying on a small sample size, which often lacks significance. Firstly, small samples tend to yield inaccurate results as they may not adequately represent the entire dataset, leading to skewed and unrealistic outcomes.
Secondly, even if results appear accurate with a small sample, generalizing them to the larger dataset becomes challenging. Sufficient sample size is necessary for reliable generalization of findings.
Lastly, small samples hinder the discovery of patterns and trends present in the original dataset. Limited data may fail to capture most of the patterns and trends, impeding comprehensive analysis.
For instance, observing only 10 customers may obscure discernible differences in purchasing patterns across age groups.
Therefore, selecting an appropriate and sizable sample, such as 200, 500, or 1,000 customers, is imperative to effectively identify patterns and trends within the data. In general, larger sample sizes yield more accurate and generalizable results, enhancing the quality of analysis.
6. Overlooking the Importance of Data Privacy
Data privacy holds paramount importance in our contemporary era, and any disregard or violation of privacy can lead to severe consequences for companies, including legal penalties, reputational damage, and financial losses. When collecting customer data, companies must prioritize ethical and responsible data processing.
Failing to inform customers about data collection or its misuse not only jeopardizes customer privacy but also tarnishes the organization’s reputation and legal standing. Research indicates that neglecting data privacy may prompt customers to disengage, with a significant portion willing to abandon a brand if their data is mishandled. Thus, it’s crucial for companies to transparently communicate, obtain explicit consent, and safeguard their customers’ data to avert potential adverse impacts.
7. Lacking Continuity of Analysis and Limiting Analysis to One-Time-Only
Analyzing customer data at a single point in time can detrimentally affect the accuracy of predicting customer churn and, consequently, the ability to proactively prevent it. Effective prediction of customer departure relies heavily on studying their behavioral patterns and trends over time, enabling companies to anticipate churn early and take preventive measures.
However, restricting analysis to a single moment in time may overlook crucial information regarding past customer behaviors that contributed to the current scenario. This limitation can lead to erroneous conclusions and inadequate predictions of customer churn risk. Analyzing data from a specific period, such as one month, fails to provide a complete and precise understanding of customer behavior as preferences and behaviors evolve over time.
For instance, a previously satisfied customer who recently started expressing dissatisfaction might go unnoticed if only one month’s data is considered. Without tracking changes in behavior over time, companies may struggle to discern the reasons behind customer dissatisfaction.
Furthermore, lacking historical data on customer behavior can obscure trends and factors influencing customer attrition, impeding efforts to retain them. Consequently, there is a pressing need for comprehensive analyses spanning different timeframes and encompassing both quantitative and qualitative data to accurately predict customer churn and devise effective retention strategies.
How can Lucidya help you avoid these seven mistakes?
Effective customer data analysis hinges on avoiding common pitfalls, and Lucidya offers valuable assistance in this regard by:
- Ensuring the collection of sufficient and accurate data: Lucidya offers tools like surveys and social listening capabilities to gather high-quality data from customers.
- Lucidya employs AI algorithms to detect and rectify biases within datasets.
- Lucidya contextualizes data analysis, leading to more precise insights and a deeper understanding of the data’s relevance within the specific industry and market.
- Lucidya furnishes comprehensive reports that reveal correlations and trends in the data, minimizing the risk of drawing erroneous conclusions.
to avoid Falling in like This is amazing Errors now And get on Visions correct with Lucidya
Conclusion
Absolutely, customer data analysis transcends mere technicality; it’s a strategic endeavor demanding profound comprehension of the business and market dynamics. It necessitates steering clear of the errors outlined in the article and beyond.
By sidestepping such pitfalls, companies can conduct more precise and effective analyses, unearthing profound insights pivotal for strategic decision-making. These insights, in turn, foster enhancements in customer experience, amplifying satisfaction levels, and fortifying brand loyalty.